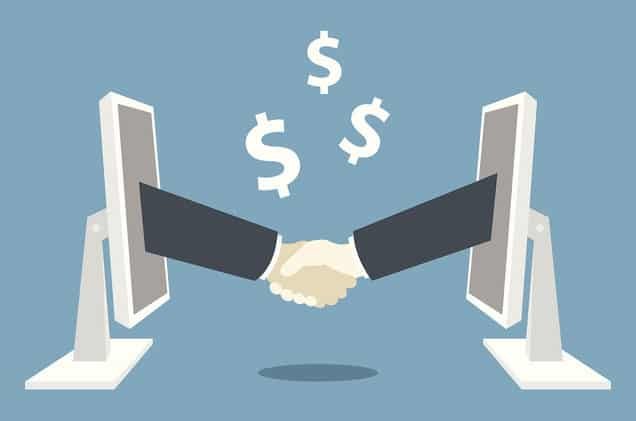
Machine learning system detects disease 95% of the time
Researchers at the University of Florida (UF) are deploying smart technology against powdery mildew, a notorious plant disease that reduces yields. In a new study, scientists used a sensing system attached to drones to collect spectral data of powdery mildew on summer squash in the fields and labs of the UF and Institute of Food and Agricultural Sciences (IFAS), Southwest Florida Research and Education Center in Immokalee, in Collier County, Florida.
“The ideal environment for powdery mildew to infect is humid weather, high-density planting and shade,” said Yiannis Ampatzidis, a UF/IFAS Assistant Professor of Agricultural and Biological Engineering as well as co-author of the study recently published in the journal Biosystems Engineering.
The main symptoms of powdery mildew are white spots or patches, usually on the leaves. Diagnosing powdery mildew in early infection stages is difficult because of symptoms on lower, more mature leaves that are often covered by other leaves.
Ampatzidis said that researchers used machine learning that can ’learn’ from spectral data to detect powdery mildew. The data came from drones and ground-based sensing systems. The trained machine-learning model identified powdery mildew in different disease development stages. The machine-learning system builds a mathematical model to detect powdery mildew without being programmed by a human to follow specific steps. Scientists detected powdery mildew about 95 percent of the time. Even without visible symptoms of the disease, the technology showed researchers the disease 82 to 89 percent of the time.